19 May 2022
Apache YuniKorn A Kubernetes Scheduler Plugin for Batch Workloads - Wilfred Spiegelenburg, Cloudera & Craig Condit, Cloudera
Kubernetes has historically focused on service-type workloads. Stateful workloads have also become better supported in recent releases. Batch scheduling continues to lag in Kubernetes core. To better support batch scheduling, several alternative schedulers have been created, including Apache YuniKorn, which has a growing community and is utilised by several large organisations such as Alibaba, Apple, and Cloudera. Over the past few years, Apache YuniKorn has matured into a highly performant, flexible workload scheduler. Recently, we have enhanced Apache YuniKorn with a new execution mode which allows Apache YuniKorn's full power and flexibility to be deployed as a set of plugins to the default Kubernetes scheduler. This allows service and batch workloads to coexist seamlessly. This session will dive into using Apache YuniKorn to schedule batch workloads leveraging the advanced options like workload queueing and quota sharing without affecting the traditional non batch Kubernetes workloads.
Kubernetes has historically focused on service-type workloads. Stateful workloads have also become better supported in recent releases. Batch scheduling continues to lag in Kubernetes core. To better support batch scheduling, several alternative schedulers have been created, including Apache YuniKorn, which has a growing community and is utilised by several large organisations such as Alibaba, Apple, and Cloudera. Over the past few years, Apache YuniKorn has matured into a highly performant, flexible workload scheduler. Recently, we have enhanced Apache YuniKorn with a new execution mode which allows Apache YuniKorn's full power and flexibility to be deployed as a set of plugins to the default Kubernetes scheduler. This allows service and batch workloads to coexist seamlessly. This session will dive into using Apache YuniKorn to schedule batch workloads leveraging the advanced options like workload queueing and quota sharing without affecting the traditional non batch Kubernetes workloads.
- 4 participants
- 30 minutes

19 May 2022
Closing - Aldo Culquicondor, Kubernetes Batch + HPC Day Program Committee Member
- 1 participant
- 3 minutes

19 May 2022
Efficient Deep Learning Training with Ludwig AutoML, Ray, and Nodeless Kubernetes - Anne Marie Holler, Elotl & Travis Addair, Predibase
Deep Learning(DL) has been successfully applied to many fields, including computer vision, natural language, business, and science. The open-source platforms Ray and Ludwig make DL accessible to diverse users, by reducing complexity barriers to training, scaling, deploying, and serving DL models. However, DL’s cost and operational overhead present significant challenges. DL model dev/test/tuning requires intermittent use of substantial GPU resources, which cloud vendors are well-positioned to provide, though at non-trivial prices. Given the expense, managing GPU resources is critical to the practical use of DL. This talk describes running Ray and Ludwig on cloud Kubernetes clusters, using Nodeless K8s to add right-sized GPU resources when they are needed and to remove them when not. Experiments comparing cost and operational overhead of using Nodeless K8s vs directly on EC2 show sizable improvements in efficiency and usability.
Deep Learning(DL) has been successfully applied to many fields, including computer vision, natural language, business, and science. The open-source platforms Ray and Ludwig make DL accessible to diverse users, by reducing complexity barriers to training, scaling, deploying, and serving DL models. However, DL’s cost and operational overhead present significant challenges. DL model dev/test/tuning requires intermittent use of substantial GPU resources, which cloud vendors are well-positioned to provide, though at non-trivial prices. Given the expense, managing GPU resources is critical to the practical use of DL. This talk describes running Ray and Ludwig on cloud Kubernetes clusters, using Nodeless K8s to add right-sized GPU resources when they are needed and to remove them when not. Experiments comparing cost and operational overhead of using Nodeless K8s vs directly on EC2 show sizable improvements in efficiency and usability.
- 5 participants
- 28 minutes
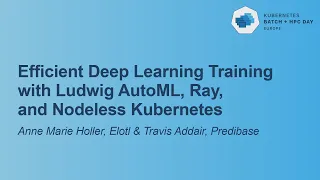
19 May 2022
Fast Data on-Ramp with Apache Pulsar on K8 - Timothy Spann, StreamNative
As the Apache Pulsar communities grows, more and more connectors will be added. To enhance the availability of sources and sinks and to make use of the greater Apache Streaming community, joining forces between Apache NiFi and Apache Pulsar is a perfect fit.
Apache NiFi also adds the benefits of ELT, ETL, data crunching, transformation, validation and batch data processing. Once data is ready to be an event, NiFi can launch it into Pulsar at light speed. I will walk through how to get started, some use cases and demos and answer questions. Benefits to the Ecosystem.
https://www.datainmotion.dev/
https://github.com/tspannhw
As the Apache Pulsar communities grows, more and more connectors will be added. To enhance the availability of sources and sinks and to make use of the greater Apache Streaming community, joining forces between Apache NiFi and Apache Pulsar is a perfect fit.
Apache NiFi also adds the benefits of ELT, ETL, data crunching, transformation, validation and batch data processing. Once data is ready to be an event, NiFi can launch it into Pulsar at light speed. I will walk through how to get started, some use cases and demos and answer questions. Benefits to the Ecosystem.
https://www.datainmotion.dev/
https://github.com/tspannhw
- 2 participants
- 14 minutes
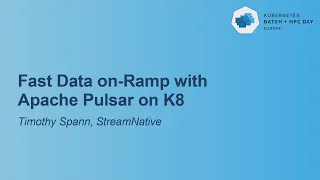
19 May 2022
Get More Computing Power by Helping the OS Scheduler - Antti Kervinen, Intel & Alexander Kanevskiy, Intel
When Linux schedules a thread on a CPU core, there is no guarantee which memories the thread will access. If the workload is lucky, the thread will use data that is already in CPU caches or in a memory that is close to the CPU core. But if not, millions of memory operations need to travel a longer way to reach the physical memory. Yet this may sound too low-level to be controllable and make a difference, you can easily help the scheduler running Kubernetes workloads, and make a big difference! Antti and Sasha show how to get a lot more computing power out of your CPUs by adding CRI Resource Manager (CRI-RM) on your Kubernetes nodes. CRI-RM affects process scheduling and memory locality by dynamically managing CPU and memory pinning of all Kubernetes containers on the node. In case studies CRI-RM has given major improvements in database and AI training performances without any workload-specific configurations or changes to upstream Kubernetes components.
When Linux schedules a thread on a CPU core, there is no guarantee which memories the thread will access. If the workload is lucky, the thread will use data that is already in CPU caches or in a memory that is close to the CPU core. But if not, millions of memory operations need to travel a longer way to reach the physical memory. Yet this may sound too low-level to be controllable and make a difference, you can easily help the scheduler running Kubernetes workloads, and make a big difference! Antti and Sasha show how to get a lot more computing power out of your CPUs by adding CRI Resource Manager (CRI-RM) on your Kubernetes nodes. CRI-RM affects process scheduling and memory locality by dynamically managing CPU and memory pinning of all Kubernetes containers on the node. In case studies CRI-RM has given major improvements in database and AI training performances without any workload-specific configurations or changes to upstream Kubernetes components.
- 3 participants
- 20 minutes
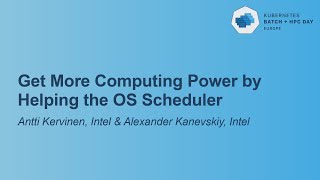
19 May 2022
How to Handle Fair Scheduling in a Private Academic K8s infrastructure - Lukas Hejtmanek, Masaryk University & Dalibor Klusacek, CESNET
While the usefulness of container-oriented computing is widely recognized, its adoption in academic environments is not so straightforward. Existing orchestrators like Kubernetes are not primarily designed to support fair execution of (bursty) workloads belonging to various researchers and/or competing projects. While public providers are using efficient pay-per-use model, academic use-cases often expect traditional fair-sharing mechanism which is widely available in current HPC installations. This talk will discuss the challenges related to the application of containerized computing within K8s-operated infrastructure used by various users and research groups in the CERIT-SC infrastructure. Specifically, we will discuss how CERIT-SC guarantees that eligible pods will be executed in a reasonable time frame, making sure that running pods of other users will eventually free their allocations to guarantee fair use of available resources.
While the usefulness of container-oriented computing is widely recognized, its adoption in academic environments is not so straightforward. Existing orchestrators like Kubernetes are not primarily designed to support fair execution of (bursty) workloads belonging to various researchers and/or competing projects. While public providers are using efficient pay-per-use model, academic use-cases often expect traditional fair-sharing mechanism which is widely available in current HPC installations. This talk will discuss the challenges related to the application of containerized computing within K8s-operated infrastructure used by various users and research groups in the CERIT-SC infrastructure. Specifically, we will discuss how CERIT-SC guarantees that eligible pods will be executed in a reasonable time frame, making sure that running pods of other users will eventually free their allocations to guarantee fair use of available resources.
- 1 participant
- 7 minutes

19 May 2022
Keynote: High Performance Computing on Google Kubernetes Engine - Maciek Różacki, Google Cloud
Google Kubernetes Engine is already a platform of choice for highly demanding high-performance computing workloads. We will present how we're investing into pushing the capabilities of our product further to maximize users' scientific output with ease, cost efficiency and industry leading performance.
Google Kubernetes Engine is already a platform of choice for highly demanding high-performance computing workloads. We will present how we're investing into pushing the capabilities of our product further to maximize users' scientific output with ease, cost efficiency and industry leading performance.
- 1 participant
- 7 minutes
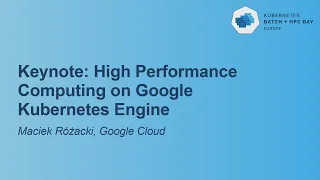
19 May 2022
Kueue: A Kubernetes-native Job Queueing - Abdullah Gharaibeh, Google
Most Kubernetes core components are pod centric, including the scheduler and cluster autoscaler. This works well for service workloads where the pods of a service are mostly independent and all services are expected to be running at all times. However, for batch workloads, it does not make sense to focus only on pods, as the partial execution of pods from multiple parallel batch jobs may lead to deadlocks where many jobs may be simultaneously active while none is able to make sufficient progress to completion or start at all. Even for single-pod batch jobs, whether on-prem or in the cloud with autoscaling capabilities, the reality is that clusters have finite capacity: constraints on resource usage exist for quota and cost management (especially true for GPUs) and so users will want an easy way to fairly and efficiently share the resources. Kueue addresses the above limitations, offering queueing capabilities commonly exist in legacy batch schedulers in the most k8s native way. It is a k8s subproject currently under development at https://github.com/kubernetes-sigs/kueue.
Most Kubernetes core components are pod centric, including the scheduler and cluster autoscaler. This works well for service workloads where the pods of a service are mostly independent and all services are expected to be running at all times. However, for batch workloads, it does not make sense to focus only on pods, as the partial execution of pods from multiple parallel batch jobs may lead to deadlocks where many jobs may be simultaneously active while none is able to make sufficient progress to completion or start at all. Even for single-pod batch jobs, whether on-prem or in the cloud with autoscaling capabilities, the reality is that clusters have finite capacity: constraints on resource usage exist for quota and cost management (especially true for GPUs) and so users will want an easy way to fairly and efficiently share the resources. Kueue addresses the above limitations, offering queueing capabilities commonly exist in legacy batch schedulers in the most k8s native way. It is a k8s subproject currently under development at https://github.com/kubernetes-sigs/kueue.
- 7 participants
- 35 minutes
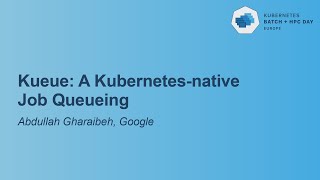
19 May 2022
Opening + Welcome - Abdullah Gharaibeh & Ricardo Rocha, Kubernetes Batch + HPC Day Program Committee Members
- 2 participants
- 6 minutes
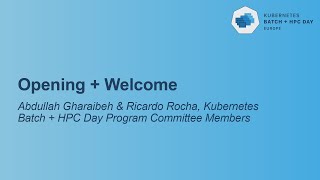
19 May 2022
Resource Orchestration of HPC on Kubernetes: Where We Are Now and the Journey Ahead! - Swati Sehgal & Francesco Romani, Red Hat
Kubernetes has become a norm for orchestrating containerized microservice applications in the domain of cloud and enterprise; it is however not yet widely adopted in HPC. HPC enablement on Kubernetes is still a challenge due to requirements like NUMA aware scheduling, advanced resource reservation/allocation capabilities and managing job dependencies and synchronization. Resource managers in Kubelet facilitate the allocation and NUMA alignment of CPU, memory, and devices. The information disconnect between kubelet and the scheduler however, is still a gap that needs to be addressed. The scheduler is oblivious to the resources availability at a more granular, NUMA-zone level which can lead to suboptimal scheduling decisions placing workloads to nodes where alignment of resources is impossible. Contributors from sig-node formed a team to address this problem and implement a numa-aware scheduler and the related infrastructure. Representing the team, the presenters will educate the attendees about the journey of this feature, challenges encountered, end to end solution, current adoption, its roadmap and cover the deployment steps for optimized performance of workloads.
Kubernetes has become a norm for orchestrating containerized microservice applications in the domain of cloud and enterprise; it is however not yet widely adopted in HPC. HPC enablement on Kubernetes is still a challenge due to requirements like NUMA aware scheduling, advanced resource reservation/allocation capabilities and managing job dependencies and synchronization. Resource managers in Kubelet facilitate the allocation and NUMA alignment of CPU, memory, and devices. The information disconnect between kubelet and the scheduler however, is still a gap that needs to be addressed. The scheduler is oblivious to the resources availability at a more granular, NUMA-zone level which can lead to suboptimal scheduling decisions placing workloads to nodes where alignment of resources is impossible. Contributors from sig-node formed a team to address this problem and implement a numa-aware scheduler and the related infrastructure. Representing the team, the presenters will educate the attendees about the journey of this feature, challenges encountered, end to end solution, current adoption, its roadmap and cover the deployment steps for optimized performance of workloads.
- 2 participants
- 26 minutes
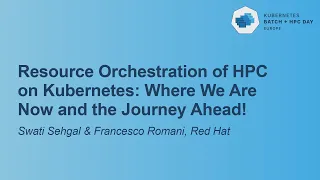
19 May 2022
Volcano – Cloud Native Batch System for AI, BigData and HPC - William(LeiBo) Wang, Huawei Cloud Computing Co., Ltd
Volcano is a cloud native batch system which is also the first batch computing project in CNCF. The major use cases are in the field of high-performance computing (HPC), such as big data, AI, Gene computing. Volcano offers job based fair-share, priority, preemption, reclaim, queue management abilities which are important for HPC users. It has integrated with computing ecosystem like spark-operator, fink-operator, kubeflow, Cromwell in big data, AI and HPC computing domains. This year Volcano is also being integrated to spark with it's custom batch scheduler natively. And many new features are being developed by contributors. f.g. co-location, elastic training, vGPU, throughput optimization and multi-cluster scheduling for HPC users.
The community has helped more than 50 users to deploy Volcano in their production environments around the world since it is open-sourced in 2019. William(Leibo) Wang who is the tech lead of Volcano community will present the latest features, use cases, progress, roadmap and best practices. He will also show how to accelerate AI training, serving, big data analysis and how to improve cluster utilization based on Volcano and other cloud native projects for users.
Volcano is a cloud native batch system which is also the first batch computing project in CNCF. The major use cases are in the field of high-performance computing (HPC), such as big data, AI, Gene computing. Volcano offers job based fair-share, priority, preemption, reclaim, queue management abilities which are important for HPC users. It has integrated with computing ecosystem like spark-operator, fink-operator, kubeflow, Cromwell in big data, AI and HPC computing domains. This year Volcano is also being integrated to spark with it's custom batch scheduler natively. And many new features are being developed by contributors. f.g. co-location, elastic training, vGPU, throughput optimization and multi-cluster scheduling for HPC users.
The community has helped more than 50 users to deploy Volcano in their production environments around the world since it is open-sourced in 2019. William(Leibo) Wang who is the tech lead of Volcano community will present the latest features, use cases, progress, roadmap and best practices. He will also show how to accelerate AI training, serving, big data analysis and how to improve cluster utilization based on Volcano and other cloud native projects for users.
- 1 participant
- 24 minutes
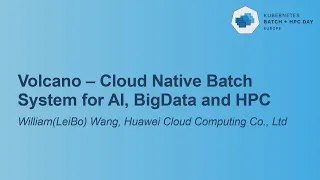