23 Jun 2022
The salinization map of the region of Flanders, Belgium shows the depth of the interface between fresh and salt groundwater in the coastal and polder area. It serves as an exploratory tool to examine the potential of groundwater projects that improve freshwater availability in the shallow subsurface. Flanders environment agency published an updated map in 2019, based on airborne time-domain electromagnetic induction data. The result of the inversion is, however, overly smooth, which potentially conceals interesting features.
Via an inverse problem, the electromagnetic induction data can be mapped onto a conductivity profile, which serves as a proxy for salinity via petrophysical laws. The inverse problem is ill-posed and regularization improves the stability of the inversion. Based on Occam’s razor principle, a smoothing constraint is typically used with a very large number of thin layers. However, the salinity profiles in the Belgian coastal plains are sometimes sharp, impeding the correct estimation of the fresh-saltwater interface. In practice, the real underground might be either blocky or smooth, or somewhere in between. Standard constraints are thus not always appropriate.
With our novel wavelet-based inversion scheme, the original time-domain AEM data can be re-interpreted in a flexible fashion, meaning that we can easily generate an ensemble of inversion models with different types of Occam's razor minimum-structure. The flexibility is due to the wavelet basis. In simple terms, a wavelet function can be seen as a building block and a simple model is one that can be built with few building blocks of various sizes. Our proposed inversion scheme adds a regularization term that limits the number of building blocks in the wavelet-domain to make sure only the necessary complexity is retrieved. The scale-dependency makes the use of small blocks more expensive, as it corresponds to adding detail. The scheme is tuned by only one additional parameter (which determines the wavelet basis function) and can recover blocky, intermediate and smooth structures. It is also capable to recover high amplitude anomalies in combination with globally smooth profiles, a common problem for smooth inversion, and an essential feature to accurately predict the salinity.
We first demonstrated this alternative inversion scheme on 1D FDEM data and now extend it to 2D AEM data a in a saltwater intrusion context . The flexibility of the method allows for choosing the appropriate sharpness for each orientation. In Figure 1, an inversion model is shown with a relatively sharp wavelet basis (db3) in the vertical orientation, while a smoother wavelet basis (db8) is used along with the lateral orientation. Imposing the appropriate sharpness on the inversion model is crucial to obtaining a reliable estimation of the fresh-saltwater interface.
The code behind the 1D regularization has been adjusted to the SimPEG framework and is open to everyone . This allows to use this flexible regularization term for many types of inversion problems and promotes geoscience reproducibility!
Bio
Wouter Deleersnyder is a passionate Ph.D. candidate at the physics department of KU Leuven and Geology department of Ghent University. With his technical skills from his physics education (Master in Physics, KU Leuven, Belgium in 2019), he wants to tackle the problems of today. Stimulated by the importance of water for man and nature, he came into contact with groundwater and geophysics. He is working on new methods to get a better picture of what is under the ground. He is specializing in electromagnetic induction surveys, forward modelling, inversion, and uncertainty quantification.
Via an inverse problem, the electromagnetic induction data can be mapped onto a conductivity profile, which serves as a proxy for salinity via petrophysical laws. The inverse problem is ill-posed and regularization improves the stability of the inversion. Based on Occam’s razor principle, a smoothing constraint is typically used with a very large number of thin layers. However, the salinity profiles in the Belgian coastal plains are sometimes sharp, impeding the correct estimation of the fresh-saltwater interface. In practice, the real underground might be either blocky or smooth, or somewhere in between. Standard constraints are thus not always appropriate.
With our novel wavelet-based inversion scheme, the original time-domain AEM data can be re-interpreted in a flexible fashion, meaning that we can easily generate an ensemble of inversion models with different types of Occam's razor minimum-structure. The flexibility is due to the wavelet basis. In simple terms, a wavelet function can be seen as a building block and a simple model is one that can be built with few building blocks of various sizes. Our proposed inversion scheme adds a regularization term that limits the number of building blocks in the wavelet-domain to make sure only the necessary complexity is retrieved. The scale-dependency makes the use of small blocks more expensive, as it corresponds to adding detail. The scheme is tuned by only one additional parameter (which determines the wavelet basis function) and can recover blocky, intermediate and smooth structures. It is also capable to recover high amplitude anomalies in combination with globally smooth profiles, a common problem for smooth inversion, and an essential feature to accurately predict the salinity.
We first demonstrated this alternative inversion scheme on 1D FDEM data and now extend it to 2D AEM data a in a saltwater intrusion context . The flexibility of the method allows for choosing the appropriate sharpness for each orientation. In Figure 1, an inversion model is shown with a relatively sharp wavelet basis (db3) in the vertical orientation, while a smoother wavelet basis (db8) is used along with the lateral orientation. Imposing the appropriate sharpness on the inversion model is crucial to obtaining a reliable estimation of the fresh-saltwater interface.
The code behind the 1D regularization has been adjusted to the SimPEG framework and is open to everyone . This allows to use this flexible regularization term for many types of inversion problems and promotes geoscience reproducibility!
Bio
Wouter Deleersnyder is a passionate Ph.D. candidate at the physics department of KU Leuven and Geology department of Ghent University. With his technical skills from his physics education (Master in Physics, KU Leuven, Belgium in 2019), he wants to tackle the problems of today. Stimulated by the importance of water for man and nature, he came into contact with groundwater and geophysics. He is working on new methods to get a better picture of what is under the ground. He is specializing in electromagnetic induction surveys, forward modelling, inversion, and uncertainty quantification.
- 5 participants
- 45 minutes

27 May 2022
With modern instrumentation time-series data is readily available heightening the level of quality control. DCIP acquisition for example, resistivities, chargeabilities and decay curves are no longer the base of validating data points. The building blocks of the calculated properties can now be analyzed to uphold a better integrity of the data itself. The challenge now is analyzing numerous time-series manually. Each point has transmit and receiver data. Particularly with distributed systems, an overwhelming amount data is available. Classically used statistical methods can be employed but can cost a lot in time. Next best would be to simulate the geophysicist looking at the data. Borrowing techniques from computer vision, convolution neural networks can be trained to “visually” inspect time-series. With most of the heavy lifting of time-series analysis alleviated, the geophysicist can have more time and information to make better informed decisions. Though DCIP time-series is the primary focus here, the techniques presented can certainly extend into time-domain controlled source electromagnetics.
Bio
John Kuttai is a senior geophysicst for DIAS Geophysical’s research and development team (also, soon to be UBC Graduate student!!). While primarily servicing the mineral exploration sector, his contributions maintain the processing and inversion needs for DC resistivity, induced polarization, magnetic gradiometry, natural and controlled source frequency domain methods. Through signal processing to inversion, a complete comprehensive work flow to manage big data and inversion for drill target identification is the main focus of his work.
Bio
John Kuttai is a senior geophysicst for DIAS Geophysical’s research and development team (also, soon to be UBC Graduate student!!). While primarily servicing the mineral exploration sector, his contributions maintain the processing and inversion needs for DC resistivity, induced polarization, magnetic gradiometry, natural and controlled source frequency domain methods. Through signal processing to inversion, a complete comprehensive work flow to manage big data and inversion for drill target identification is the main focus of his work.
- 8 participants
- 54 minutes
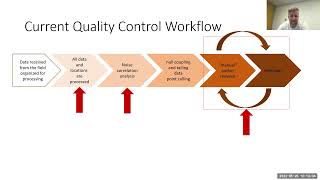
21 Apr 2022
Abstract
Scientific communication today is designed around print documents and paywalled access to content. Over the last decade, the open-science movement has accelerated the use of pre-print services and data archives that are vastly improving the accessibility of scientific content. However, these systems are not designed for communicating modern scientific outputs, which encompasses much more than a “paper-centric model of the scholarly literature”.
In this presentation, we will give a background on challenges with today’s tools for research communication & collaboration, and present a vision for the future that follows the FORCE11 recommendations (Bourne et al., 2012). Specifically: (1) rethink the unit and form of scholarly publication; (2) develop tools and technologies to better support the scholarly lifecycle; and (3) add data, software, and workflows as first-class research objects.
We will discuss these recommendations in the context of (a) the ExecutableBooks community and new markup languages for scientific communication; (b) a collaborative writing tool called Curvenote that integrates with Jupyter Notebooks, and (c) new publishing tools that support networked scientific communication throughout the scholarly lifecycle.
Excerpts from FORCE11:
“We see a future in which scientific information and scholarly communication more generally become part of a global, universal and explicit network of knowledge; where every claim, hypothesis, argument—every significant element of the discourse—can be explicitly represented, along with supporting data, software, workflows, multimedia, external commentary, and information about provenance.”“This vision moves away from the paper-centric model of the scholarly literature, towards a more distributed network-centric model” that “vastly improves knowledge transfer and [has a] far wider impact.” (Bourne et al., 2012)
Bio
Rowan completed his PhD in Geophysics at the University of British Columbia, Canada, in 2017. His PhD laid out a framework for geophysical inversions (SimPEG!) and applied it to large-scale, vadose-zone groundwater flow. Currently, Rowan works at Curvenote, a company that sits at the intersection of scientific collaboration, publishing, and technology and provides tools for writing and collaborating on technical documents. Rowan founded Curvenote in 2020, with the mission to help get scientific communication out of PDFs and onto the web — the way we communicate scientific knowledge should evolve past the status quo of print-based publishing and all the limitations of paper. He is a core team member of the ExecutableBooks community, which supports MyST Markdown and JupyterBook.
Scientific communication today is designed around print documents and paywalled access to content. Over the last decade, the open-science movement has accelerated the use of pre-print services and data archives that are vastly improving the accessibility of scientific content. However, these systems are not designed for communicating modern scientific outputs, which encompasses much more than a “paper-centric model of the scholarly literature”.
In this presentation, we will give a background on challenges with today’s tools for research communication & collaboration, and present a vision for the future that follows the FORCE11 recommendations (Bourne et al., 2012). Specifically: (1) rethink the unit and form of scholarly publication; (2) develop tools and technologies to better support the scholarly lifecycle; and (3) add data, software, and workflows as first-class research objects.
We will discuss these recommendations in the context of (a) the ExecutableBooks community and new markup languages for scientific communication; (b) a collaborative writing tool called Curvenote that integrates with Jupyter Notebooks, and (c) new publishing tools that support networked scientific communication throughout the scholarly lifecycle.
Excerpts from FORCE11:
“We see a future in which scientific information and scholarly communication more generally become part of a global, universal and explicit network of knowledge; where every claim, hypothesis, argument—every significant element of the discourse—can be explicitly represented, along with supporting data, software, workflows, multimedia, external commentary, and information about provenance.”“This vision moves away from the paper-centric model of the scholarly literature, towards a more distributed network-centric model” that “vastly improves knowledge transfer and [has a] far wider impact.” (Bourne et al., 2012)
Bio
Rowan completed his PhD in Geophysics at the University of British Columbia, Canada, in 2017. His PhD laid out a framework for geophysical inversions (SimPEG!) and applied it to large-scale, vadose-zone groundwater flow. Currently, Rowan works at Curvenote, a company that sits at the intersection of scientific collaboration, publishing, and technology and provides tools for writing and collaborating on technical documents. Rowan founded Curvenote in 2020, with the mission to help get scientific communication out of PDFs and onto the web — the way we communicate scientific knowledge should evolve past the status quo of print-based publishing and all the limitations of paper. He is a core team member of the ExecutableBooks community, which supports MyST Markdown and JupyterBook.
- 6 participants
- 54 minutes

24 Mar 2022
Abstract
The use of airborne electromagnetic (AEM) data for geoscience applications is rapidly increasing. For instance, in California USA, there is an ongoing AEM project led by the California Department of Water Resources (CDWR), which plans to map out most of the Central Valley of California and some water basins in California. All acquired AEM data and resulting interpretation of the data, which are resistivity models of the subsurface will be publicly available. There are many big initiatives like this project throughout the world. Therefore, it is critical to understand how the resulting resistivity models are obtained from the acquired AEM data, and further equipped with an ability to download and explore the available resistivity data. In this talk, I will first introduce how a resistivity model is obtained from the AEM data then introduce open-source tools that can be used to explore this resistivity model.
Bio
Seogi completed his PhD in Geophysics at the University of British Columbia, Canada, in 2018. His thesis work focused on computational electromagnetics and its application to mining problems. Currently, he is a Postdoctoral Researcher in the Geophysics Department at Stanford. His research focus is on advancing the use of airborne electromagnetic and other remote sensing methods for groundwater management and groundwater science. He continues to contribute to the development of open source software, SimPEG, and educational resources, GeoSci.xyz, for geophysics.
The use of airborne electromagnetic (AEM) data for geoscience applications is rapidly increasing. For instance, in California USA, there is an ongoing AEM project led by the California Department of Water Resources (CDWR), which plans to map out most of the Central Valley of California and some water basins in California. All acquired AEM data and resulting interpretation of the data, which are resistivity models of the subsurface will be publicly available. There are many big initiatives like this project throughout the world. Therefore, it is critical to understand how the resulting resistivity models are obtained from the acquired AEM data, and further equipped with an ability to download and explore the available resistivity data. In this talk, I will first introduce how a resistivity model is obtained from the AEM data then introduce open-source tools that can be used to explore this resistivity model.
Bio
Seogi completed his PhD in Geophysics at the University of British Columbia, Canada, in 2018. His thesis work focused on computational electromagnetics and its application to mining problems. Currently, he is a Postdoctoral Researcher in the Geophysics Department at Stanford. His research focus is on advancing the use of airborne electromagnetic and other remote sensing methods for groundwater management and groundwater science. He continues to contribute to the development of open source software, SimPEG, and educational resources, GeoSci.xyz, for geophysics.
- 7 participants
- 55 minutes
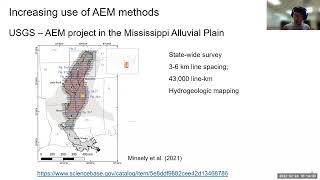
18 Feb 2022
Randomize-then-optimize: nonlinear uncertainty quantification for regularized inversion
Much of our understanding of the Earth's subsurface comes from physical models produced by inverting geophysical observations made at the surface. Inversion is non-unique and nonlinear, however, meaning there is significant uncertainty in the inverted model parameters. The standard inversion method in geophysics remains regularized inversion despite the fact that it produces single model estimates without a meaningful way to quantify the uncertainty. In this talk I will present 'randomize-then-optimize' (RTO), an uncertainty quantification (UQ) strategy for regularized inversion. This method reinterprets regularized inversion in a Bayesian context, turning these familiar algorithms into Bayesian samplers capable of producing model uncertainty efficiently, even for large geophysical problems. I will discuss the basic theory behind RTO, describe our extension of it to hierarchically sample the regularization strength (which we call RTO-TKO), and show results on field data examples from electromagnetic geophysics.
Bio
Daniel is the John W Miles postdoctoral scholar in computational and theoretical geophysics at the Scripps Institution of Oceanography, UC San Diego. His research interests are primarily focused in two fields: (1) the lithosphere-asthenosphere system, and the role of fluids in particular, and their relation to plate tectonics; and (2) the development of algorithms capable of quantifying uncertainty in inverted subsurface models inferred from geophysical data. He studied computational mathematics at Stanford University, receiving a Masters in 2015. He did his doctoral studies in geophysics with professor Kerry Key at Columbia University, receiving a PhD in 2020.
Much of our understanding of the Earth's subsurface comes from physical models produced by inverting geophysical observations made at the surface. Inversion is non-unique and nonlinear, however, meaning there is significant uncertainty in the inverted model parameters. The standard inversion method in geophysics remains regularized inversion despite the fact that it produces single model estimates without a meaningful way to quantify the uncertainty. In this talk I will present 'randomize-then-optimize' (RTO), an uncertainty quantification (UQ) strategy for regularized inversion. This method reinterprets regularized inversion in a Bayesian context, turning these familiar algorithms into Bayesian samplers capable of producing model uncertainty efficiently, even for large geophysical problems. I will discuss the basic theory behind RTO, describe our extension of it to hierarchically sample the regularization strength (which we call RTO-TKO), and show results on field data examples from electromagnetic geophysics.
Bio
Daniel is the John W Miles postdoctoral scholar in computational and theoretical geophysics at the Scripps Institution of Oceanography, UC San Diego. His research interests are primarily focused in two fields: (1) the lithosphere-asthenosphere system, and the role of fluids in particular, and their relation to plate tectonics; and (2) the development of algorithms capable of quantifying uncertainty in inverted subsurface models inferred from geophysical data. He studied computational mathematics at Stanford University, receiving a Masters in 2015. He did his doctoral studies in geophysics with professor Kerry Key at Columbia University, receiving a PhD in 2020.
- 9 participants
- 1:20 hours
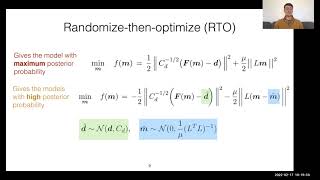
24 Jan 2022
With distributed array 3D DCIP aquisition and DIAS’s Common Voltage Reference method, the number of possible dipole collection grows signficantly compared to traditional 2D. Data sets can easily grow into a million or more data points. Being distributed, aquisition over extreme topography is done frequently. This often leads to large mesh cell counts for the fine discretization of the topography. The combination of numerous transmits and large cell counts heavily consume computational resources and take significant time for inversion. To improve performance, parallelizing the inversion is required. Configuring a cluster with the right combination of hardware and software can provide significant gains. SimPEG code is then modified to divide the inversion problem into smaller simulations which are assigned to each cluster node. Here I explore inverting datasets from small to large data and cell counts. The largest requiring more than 1TB of RAM, which is larger than most workstation’s capacity. The results and the work are based on the SimPEG framework parallelized for effective cluster usage.
Bio
John Kuttai is a senior geophysicst for DIAS Geophysical’s research and development team. While primarily servicing the mineral exploration sector, his contributions maintain the processing and inversion needs for DC resistivity, induced polarization, magnetic gradiometry, natural and controlled source frequency domain methods. Through signal processing to inversion, a complete comprehensive work flow to manage big data and inversion for drill target identification is the main focus of his work.
Bio
John Kuttai is a senior geophysicst for DIAS Geophysical’s research and development team. While primarily servicing the mineral exploration sector, his contributions maintain the processing and inversion needs for DC resistivity, induced polarization, magnetic gradiometry, natural and controlled source frequency domain methods. Through signal processing to inversion, a complete comprehensive work flow to manage big data and inversion for drill target identification is the main focus of his work.
- 7 participants
- 49 minutes
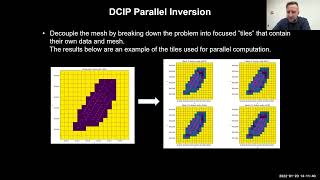
20 Nov 2021
Cones, calderas and fields (volcanic and potential). Imaging the volcano factory
Interpretation and inversion of potential field data offer the abiity to image in high resolution shallow and mid crustal structures, including those caused by magmatism and volcanism. Here I present examples from three volcanic regions in Chile and New Zealand. At Laguna del Maule Volcanic Field, Chile, gravity inversion reveals a low density body at 2 km depth, interpreted by use of thermodynamic models as a high silica rhyolite magma with a free volatile content. At Okataina Volcanic Center, interpretation and inversion of new and existing terrestrial, lake and airborne gravity and magnetic data reveals the evolution of the caldera through repeated episodes of eruption, collapse and infill. At Mt. Ruapehu, New Zealand, inversion of helimag data in conjunction with interpretation of hyperspectral imaging maps the distribution of surface alteration and its volumetric extent with implications for probability of future large scale flank collapse. All models presented rely on the SimPEG inversion framework which is and continues to be a critical tool for my work.
Craig Miller is a senior volcano geophysicist at GNS Science New Zealand specialising in imaging volcano, magmatic and hydrothermal systems using potential field geophysical methods, such as gravity, magnetics and resistivity. Through inverse and forward models these data are turned into images of the Earth’s crust to locate features of interest. He has a broad background in geosciences having worked in mineral exploration, volcano monitoring and geothermal assessment.
Interpretation and inversion of potential field data offer the abiity to image in high resolution shallow and mid crustal structures, including those caused by magmatism and volcanism. Here I present examples from three volcanic regions in Chile and New Zealand. At Laguna del Maule Volcanic Field, Chile, gravity inversion reveals a low density body at 2 km depth, interpreted by use of thermodynamic models as a high silica rhyolite magma with a free volatile content. At Okataina Volcanic Center, interpretation and inversion of new and existing terrestrial, lake and airborne gravity and magnetic data reveals the evolution of the caldera through repeated episodes of eruption, collapse and infill. At Mt. Ruapehu, New Zealand, inversion of helimag data in conjunction with interpretation of hyperspectral imaging maps the distribution of surface alteration and its volumetric extent with implications for probability of future large scale flank collapse. All models presented rely on the SimPEG inversion framework which is and continues to be a critical tool for my work.
Craig Miller is a senior volcano geophysicist at GNS Science New Zealand specialising in imaging volcano, magmatic and hydrothermal systems using potential field geophysical methods, such as gravity, magnetics and resistivity. Through inverse and forward models these data are turned into images of the Earth’s crust to locate features of interest. He has a broad background in geosciences having worked in mineral exploration, volcano monitoring and geothermal assessment.
- 7 participants
- 1:10 hours
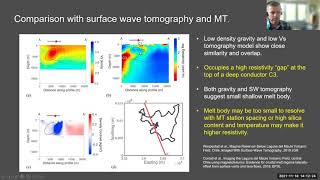
14 Oct 2021
Investigating the recoverability of physical property relationships from geophysical inversions of multiple potential-field data
Xinyan Li and Jiajia Sun
Collecting and interpreting multiple potential-field datasets have been popular in resource explorations because potential-field data contains valuable information about subsurface structures and compositions. The general interpretation workflow involves two steps. In the first step, potential-field data sets are inverted, either separately or jointly, to obtain multiple physical property models. In the second step, a process called geology differentiation is typically applied where inverted physical property values are classified into distinct classes. The implicit assumption here is that the recovered physical property relationships from geophysical inversions are reliable. However, whether this assumption holds true or not remains to be investigated. Thus, our first research question is: (1) under what conditions would the recovered physical property relationships become unreliable? Moreover, it is well known that the standard L2-norm inversions would underestimate physical property values. How the underestimation affects the recoverability is underexplored. On the other hand, the sparse norm regularization method has proved to be able to recover models with compact boundaries and elevated values. Therefore, our second research question is: (2) how would the sparse norm inversions affect the recoverability of physical property relationships?
To investigate the recoverability of physical property relationships and to answer these questions, we have designed six geological scenarios with causative geological units at various depths and differed in physical property magnitudes. For each scenario, we simulated gravity and magnetic data, performed separate and joint inversions in both smooth L2-norm and sparse L12-norm, and followed by geology differentiation. Our work shows that (1) the recoverability of physical property relationships is significantly affected by the depths of the source bodies, and (2) joint sparsity inversion results in the best recoverability consistently in all scenarios. Our work provides a strong motivation for implementing joint sparsity inversion when the goal is to identify different geological units based on potential-field data. We are currently applying the same workflow to the airborne gravity and magnetic data collected over the QUEST project in British Columbia of Canada, where the objective is to identify prospective areas for future mineral exploration hindered by the thick glacial cover.
Bio. Xinyan Li is pursuing her Ph.D. degree in geophysics, in the Department of Earth and Atmospheric Sciences at the University of Houston. She received a B.Sc. degree in geophysics in 2017 also from the University of Houston. Her research interests focus on maximizing the value of geophysical data through 3D mixed Lp-norm joint inversion and geology differentiation. She currently works on joint inversion and recoverability analysis using multiple potential-field data, primarily applied to mineral prospectivity mapping in the QUEST project area in British Columbia of Canada.
Xinyan Li and Jiajia Sun
Collecting and interpreting multiple potential-field datasets have been popular in resource explorations because potential-field data contains valuable information about subsurface structures and compositions. The general interpretation workflow involves two steps. In the first step, potential-field data sets are inverted, either separately or jointly, to obtain multiple physical property models. In the second step, a process called geology differentiation is typically applied where inverted physical property values are classified into distinct classes. The implicit assumption here is that the recovered physical property relationships from geophysical inversions are reliable. However, whether this assumption holds true or not remains to be investigated. Thus, our first research question is: (1) under what conditions would the recovered physical property relationships become unreliable? Moreover, it is well known that the standard L2-norm inversions would underestimate physical property values. How the underestimation affects the recoverability is underexplored. On the other hand, the sparse norm regularization method has proved to be able to recover models with compact boundaries and elevated values. Therefore, our second research question is: (2) how would the sparse norm inversions affect the recoverability of physical property relationships?
To investigate the recoverability of physical property relationships and to answer these questions, we have designed six geological scenarios with causative geological units at various depths and differed in physical property magnitudes. For each scenario, we simulated gravity and magnetic data, performed separate and joint inversions in both smooth L2-norm and sparse L12-norm, and followed by geology differentiation. Our work shows that (1) the recoverability of physical property relationships is significantly affected by the depths of the source bodies, and (2) joint sparsity inversion results in the best recoverability consistently in all scenarios. Our work provides a strong motivation for implementing joint sparsity inversion when the goal is to identify different geological units based on potential-field data. We are currently applying the same workflow to the airborne gravity and magnetic data collected over the QUEST project in British Columbia of Canada, where the objective is to identify prospective areas for future mineral exploration hindered by the thick glacial cover.
Bio. Xinyan Li is pursuing her Ph.D. degree in geophysics, in the Department of Earth and Atmospheric Sciences at the University of Houston. She received a B.Sc. degree in geophysics in 2017 also from the University of Houston. Her research interests focus on maximizing the value of geophysical data through 3D mixed Lp-norm joint inversion and geology differentiation. She currently works on joint inversion and recoverability analysis using multiple potential-field data, primarily applied to mineral prospectivity mapping in the QUEST project area in British Columbia of Canada.
- 8 participants
- 57 minutes
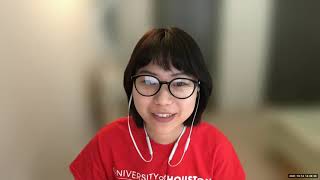
12 Aug 2021
August 2021 SimPEG Seminar. Implementing geological rules within geophysical inversion: A PGI perspective
Inferring geologically meaningful information from a geophysical inversion is a challenging task. Moreover, prior knowledge about the petrophysical contrasts or the relationships between various geological units can also prove difficult to translate into quantitative input for the inversion. In previous works, we developed a Petrophysically and Geologically guided Inversion (PGI) framework that enables desired petrophysical characteristics to be reproduced. This information is encoded into the objective function's smallness through a Gaussian Mixture Model (GMM). The resulting discrete geological representation of the subsurface thus fits both the geophysical and petrophysical information. The way we included geological information was limited to favouring the occurrence of chosen rock units in user-defined areas on a cell-by-cell approach. Transferring geological information from one area to another, such as an expected stratigraphy, was not easily done. Moreover, structural information (dip orientation, etc.), which by definition depends on multiple cells at once, was left to the objective function's smoothness, which acts on the physical property models rather than on the geological representation itself. We improve upon the existing PGI framework to make the inversion result geologically realistic by including geological rules as part of the process that builds the geological representation throughout the inversion's iterations. For this purpose, we incorporate image segmentation tools using Markov Random Field (MRF) as part of the PGI framework. The final recovered model fits geophysical and petrophysical information while reproducing geological characteristics, thus providing a more faithful and informed representation of the underground.
Thibaut Astic received a Ph.D. (2020) from the Geophysical Inversion Facility (GIF) at the University of British Columbia (Vancouver, BC, Canada), where he is currently working as a postdoctoral researcher. His research focuses on joint inversion coupled by petrophysical and geological information and the development of open-source tools for the geosciences community, mostly through the Python package SimPEG. Before his Ph.D., Thibaut worked in geological mapping and geophysical data acquisition and processing at the Quebec Department of Natural Resources and various geophysics companies.
Inferring geologically meaningful information from a geophysical inversion is a challenging task. Moreover, prior knowledge about the petrophysical contrasts or the relationships between various geological units can also prove difficult to translate into quantitative input for the inversion. In previous works, we developed a Petrophysically and Geologically guided Inversion (PGI) framework that enables desired petrophysical characteristics to be reproduced. This information is encoded into the objective function's smallness through a Gaussian Mixture Model (GMM). The resulting discrete geological representation of the subsurface thus fits both the geophysical and petrophysical information. The way we included geological information was limited to favouring the occurrence of chosen rock units in user-defined areas on a cell-by-cell approach. Transferring geological information from one area to another, such as an expected stratigraphy, was not easily done. Moreover, structural information (dip orientation, etc.), which by definition depends on multiple cells at once, was left to the objective function's smoothness, which acts on the physical property models rather than on the geological representation itself. We improve upon the existing PGI framework to make the inversion result geologically realistic by including geological rules as part of the process that builds the geological representation throughout the inversion's iterations. For this purpose, we incorporate image segmentation tools using Markov Random Field (MRF) as part of the PGI framework. The final recovered model fits geophysical and petrophysical information while reproducing geological characteristics, thus providing a more faithful and informed representation of the underground.
Thibaut Astic received a Ph.D. (2020) from the Geophysical Inversion Facility (GIF) at the University of British Columbia (Vancouver, BC, Canada), where he is currently working as a postdoctoral researcher. His research focuses on joint inversion coupled by petrophysical and geological information and the development of open-source tools for the geosciences community, mostly through the Python package SimPEG. Before his Ph.D., Thibaut worked in geological mapping and geophysical data acquisition and processing at the Quebec Department of Natural Resources and various geophysics companies.
- 7 participants
- 1:14 hours
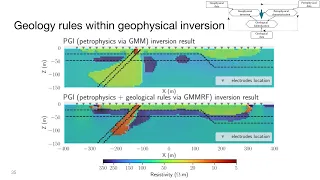
15 Jul 2021
July 2021 SimPEG Seminar. On recovering changes of water head from satellite ground deformation data
Population growth and climate change in 21 century increase the demand for groundwater threatening the sustainability of groundwater resources. Therefore, there is an urgent need to understand the groundwater system. Major drivers of the system are the spatial and temporal changes of the water head, and therefore obtaining these changes is an essential task. Although head data can be measured from water wells (e.g. monitoring, irrigation), the spatial coverage and density of the wells are often poor resulting in a large data gap between the wells. The ground deformation signals, which can be measured from satellites (e.g. Sentinel), contain information about the changes of the head providing great potential to fill in this data gap. Working on the ground deformation data and head data acquired at the Central Valley of California, which is one of the most productive farmland in the world resulting in a large amount of water demand, I present a developed methodology that can 1) simulate the deformation signals from given head data and other aquifer parameters and 2) invert the observed deformation signals to estimate head data and other aquifer parameters.
Seogi completed his PhD in Geophysics at the University of British Columbia, Canada, in 2018. His thesis work focused on computational electromagnetics and its application to mining problems. Currently, he is a Postdoctoral Researcher in the Geophysics Department at Stanford. His research focus is on advancing the use of airborne electromagnetic and other remote sensing methods for groundwater management and groundwater science. He continues to contribute to the development of open source software, SimPEG, and educational resources, GeoSci.xyz, for geophysics.
Population growth and climate change in 21 century increase the demand for groundwater threatening the sustainability of groundwater resources. Therefore, there is an urgent need to understand the groundwater system. Major drivers of the system are the spatial and temporal changes of the water head, and therefore obtaining these changes is an essential task. Although head data can be measured from water wells (e.g. monitoring, irrigation), the spatial coverage and density of the wells are often poor resulting in a large data gap between the wells. The ground deformation signals, which can be measured from satellites (e.g. Sentinel), contain information about the changes of the head providing great potential to fill in this data gap. Working on the ground deformation data and head data acquired at the Central Valley of California, which is one of the most productive farmland in the world resulting in a large amount of water demand, I present a developed methodology that can 1) simulate the deformation signals from given head data and other aquifer parameters and 2) invert the observed deformation signals to estimate head data and other aquifer parameters.
Seogi completed his PhD in Geophysics at the University of British Columbia, Canada, in 2018. His thesis work focused on computational electromagnetics and its application to mining problems. Currently, he is a Postdoctoral Researcher in the Geophysics Department at Stanford. His research focus is on advancing the use of airborne electromagnetic and other remote sensing methods for groundwater management and groundwater science. He continues to contribute to the development of open source software, SimPEG, and educational resources, GeoSci.xyz, for geophysics.
- 9 participants
- 1:05 hours
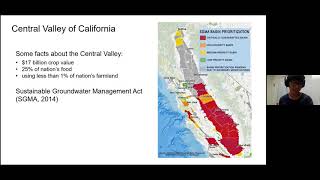