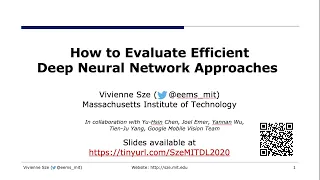
Description
Enabling the efficient processing of deep neural networks (DNNs) has becoming increasingly important to enable the deployment of DNNs on a wide range of platforms, for a wide range of applications. To address this need, there has been a significant amount of work in recent years on designing DNN accelerators and developing approaches for efficient DNN processing that spans the computer vision, machine learning, and hardware/systems architecture communities. Given the volume of work, it would not be feasible to cover them all in a single talk. Instead, this talk will focus on *how* to evaluate these different approaches, which include the design of DNN accelerators and DNN models. It will also highlight the key metrics that should be measured and compared and present tools that can assist in the evaluation.
Slides for the talk are available at https://www.rle.mit.edu/eems/publications/tutorials/
Related article available at https://www.rle.mit.edu/eems/wp-content/uploads/2020/09/ieee_mssc_summer2020.pdf
If you would like to learn more, please check out our recently published a book on "Efficient Processing of Deep Neural Networks" at https://tinyurl.com/EfficientDNNBook
Excerpts are available at http://eyeriss.mit.edu/tutorial.html
We also hold a two-day MIT Professional Education Short Course on "Designing Efficient Deep Learning Systems". Find out more at http://shortprograms.mit.edu/dls
------------
References cited in this talk
------------
* Limitations of Existing Efficient DNN Approaches
- Y.-H. Chen*, T.-J. Yang*, J. Emer, V. Sze, “Understanding the Limitations of Existing Energy-Efficient Design Approaches for Deep Neural Networks,” SysML Conference, February 2018.
- V. Sze, Y.-H. Chen, T.-J. Yang, J. Emer, “Efficient Processing of Deep Neural Networks: A Tutorial and Survey,” Proceedings of the IEEE, vol. 105, no. 12, pp. 2295-2329, December 2017.
- Hardware Architecture for Deep Neural Networks: http://eyeriss.mit.edu/tutorial.html
* Co-Design of Algorithms and Hardware for Deep Neural Networks
- T.-J. Yang, Y.-H. Chen, V. Sze, “Designing Energy-Efficient Convolutional Neural Networks using Energy-Aware Pruning,” IEEE Conference on Computer Vision and Pattern Recognition (CVPR), 2017.
Energy estimation tool: http://eyeriss.mit.edu/energy.html
- T.-J. Yang, A. Howard, B. Chen, X. Zhang, A. Go, V. Sze, H. Adam, “NetAdapt: Platform-Aware Neural Network Adaptation for Mobile Applications,” European Conference on Computer Vision (ECCV), 2018. http://netadapt.mit.edu/
* Processing In Memory
- T.-J. Yang, V. Sze, “Design Considerations for Efficient Deep Neural Networks on Processing-in-Memory Accelerators,” IEEE International Electron Devices Meeting (IEDM), Invited Paper, December 2019. http://www.rle.mit.edu/eems/wp-content/uploads/2019/12/2019_iedm_pim.pdf
* Energy-Efficient Hardware for Deep Neural Networks
Project website: http://eyeriss.mit.edu
- Y.-H. Chen, T. Krishna, J. Emer, V. Sze, “Eyeriss: An Energy-Efficient Reconfigurable Accelerator for Deep Convolutional Neural Networks,” IEEE Journal of Solid-State Circuits (JSSC), ISSCC Special Issue, Vol. 52, No. 1, pp. 127-138, January 2017.
- Y.-H. Chen, J. Emer, V. Sze, “Eyeriss: A Spatial Architecture for Energy-Efficient Dataflow for Convolutional Neural Networks,” International Symposium on Computer Architecture (ISCA), pp. 367-379, June 2016.
- Y.-H. Chen, T.-J. Yang, J. Emer, V. Sze, “Eyeriss v2: A Flexible Accelerator for Emerging Deep Neural Networks on Mobile Devices,” IEEE Journal on Emerging and Selected Topics in Circuits and Systems (JETCAS), June 2019.
- Eyexam: https://arxiv.org/abs/1807.07928
* DNN Processor Evaluation Tools
- Wu et al., “Accelergy: An Architecture-Level Energy Estimation Methodology for Accelerator Designs,” ICCAD 2019, http://accelergy.mit.edu
- Wu et al., “An Architecture-Level Energy and Area Estimator for Processing-In-Memory Accelerator Designs,” ISPASS 2020, http://accelergy.mit.edu
- Parashar et al., “Timeloop: A Systematic Approach to DNN Accelerator Evaluation,” ISPASS 2019