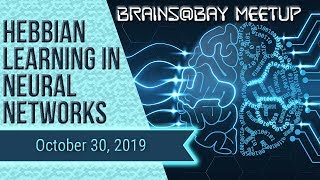
Description
https://www.meetup.com/BraIns-Bay/events/265724515/
Meeting starts at 30:00.
Welcome to another session of Brains@Bay! This time we will be talking about Hebbian Learning, the main mechanism used by the brain to learn new experiences.
As always, we will start with a perspective from neuroscience, then move on to understand how these concepts can be applied to machine learning. Our meetup is a journal club, so expect a lot of very interesting discussions during the presentations.
We've changed the location for this next event to make it more comfortable for everyone - we've partnered with UCSC and will hold the event at UCSC Silicon Valley Campus. Please see the details on the meetup page.
Agenda:
** 06:30 - 07:10: Networking and Pizza Time!
** 07:10 - 07:50: Hebbian Learning in Neuroscience - Floran Fiebig, PhD
Title: Bayesian-Hebbian learning in spike-based cortical network simulations.
Many Hebbian learning rules exist, serving a variety of local learning rules that both explain neuroscientific findings and shape various networks in machine learning. In a biophysical model of spiking cortical neural networks, we leverage abstracted processes of synaptic and nonsynaptic changes, to derive a local, online Hebbian learning rule for spiking neurons inspired by Bayesian statistics. The resulting spiking implementation of a Bayesian Confidence Propagation Neural Network (BCPNN) is capable of explaining a broad array of neuroscientific findings and perform a variety of cognitive tasks, but may also be interesting to ML investigators puzzled by the spiking nature of neuronal computation, and how one might want to approach the interesting problem of mapping existing learning rules onto a network that leverages discrete spikes as a means of information propagation and unsupervised online learning. SLIDES: https://prezi.com/view/Hf1MBr8cfXSNs8LeVDqt/
** 07:50 - 08:30: Hebbian Learning in Machine Learning - Thomas Miconi, PhD
Title: Differentiable plasticity: training self-modifying networks for fast learning with Hebbian plasticity
Neural networks are usually trained over long periods with massive amounts of data. By contrast, animals and humans can learn complex information very quickly. This ability for fast lifelong learning results from (largely Hebbian) synaptic plasticity, carefully tuned by evolution. Here we show that plastic networks can be trained to learn quickly and efficiently, using standard gradient descent instead of evolution. The trained networks can quickly learn high-dimensional information and use it to solve a task. Our results show that differentiable plasticity may provide a powerful novel approach to the learning-to-learn problem.
** 08:30 - 09:00 Discussions and Wrap-up
0:00 Starting soon...
30:51 Floran Fiebig - Hebbian Learning in Neuroscience
1:20:00 Thomas Miconi - Hebbian Learning in Machine Learning