1 Mar 2017
My guest this time is Francisco Webber, founder and General Manager of artificial intelligence startup Cortical.io. Francisco presented at the O’Reilly AI conference on an approach to natural language understanding based on semantic representations of speech. His talk was called “AI is not a matter of strength but of intelligence.” My conversation with Francisco was a bit technical and abstract, but also super interesting.
The show notes can be found at https://twimlai.com/talk/10.
Subscribe!
iTunes ➙ https://itunes.apple.com/us/podcast/this-week-in-machine-learning/id1116303051?mt=2
Soundcloud ➙ https://soundcloud.com/twiml
Google Play ➙ http://bit.ly/2lrWlJZ
Stitcher ➙ http://www.stitcher.com/s?fid=92079&refid=stpr
RSS ➙ https://twimlai.com/feed
Lets Connect!
Twimlai.com ➙ https://twimlai.com/contact
Twitter ➙ https://twitter.com/twimlai
Facebook ➙ https://Facebook.com/Twimlai
Medium ➙ https://medium.com/this-week-in-machine-learning-ai
The show notes can be found at https://twimlai.com/talk/10.
Subscribe!
iTunes ➙ https://itunes.apple.com/us/podcast/this-week-in-machine-learning/id1116303051?mt=2
Soundcloud ➙ https://soundcloud.com/twiml
Google Play ➙ http://bit.ly/2lrWlJZ
Stitcher ➙ http://www.stitcher.com/s?fid=92079&refid=stpr
RSS ➙ https://twimlai.com/feed
Lets Connect!
Twimlai.com ➙ https://twimlai.com/contact
Twitter ➙ https://twitter.com/twimlai
Facebook ➙ https://Facebook.com/Twimlai
Medium ➙ https://medium.com/this-week-in-machine-learning-ai
- 3 participants
- 49 minutes

20 May 2016
Using SDR sets and unions to identify SDRs that have been seen in the past.
Help me decide what episode to do next, Encoders or Spatial Pooling! Comment below or vote here: https://discourse.numenta.org/t/htm-school-episode-4-sdr-sets-unions/455
Intro music: "Books" by Minden: https://minden.bandcamp.com/track/books-2
Help me decide what episode to do next, Encoders or Spatial Pooling! Comment below or vote here: https://discourse.numenta.org/t/htm-school-episode-4-sdr-sets-unions/455
Intro music: "Books" by Minden: https://minden.bandcamp.com/track/books-2
- 1 participant
- 14 minutes
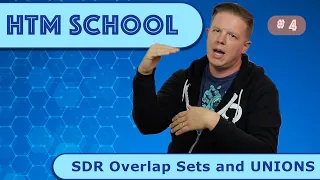
29 Apr 2016
In this episode of HTM School, we talk about SDR overlap sets and subsampling.
Properties of Sparse Distributed Representations and their Application to Hierarchical Temporal Memory: http://arxiv.org/abs/1503.07469
SDR Visualizations: https://github.com/nupic-community/sdr-viz
Intro music: "Books" by Minden: https://minden.bandcamp.com/track/books-2
Properties of Sparse Distributed Representations and their Application to Hierarchical Temporal Memory: http://arxiv.org/abs/1503.07469
SDR Visualizations: https://github.com/nupic-community/sdr-viz
Intro music: "Books" by Minden: https://minden.bandcamp.com/track/books-2
- 1 participant
- 15 minutes
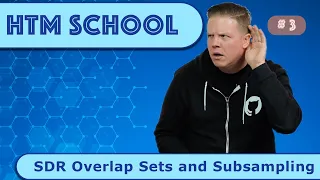
15 Apr 2016
In this episode of HTM School, we formally introduce the Sparse Distributed Representation (SDR).
Properties of Sparse Distributed Representations and their Application to Hierarchical Temporal Memory: http://arxiv.org/abs/1503.07469
SDR Visualizations: https://github.com/nupic-community/sdr-viz
Intro music: "Books" by Minden: https://minden.bandcamp.com/track/books-2
Properties of Sparse Distributed Representations and their Application to Hierarchical Temporal Memory: http://arxiv.org/abs/1503.07469
SDR Visualizations: https://github.com/nupic-community/sdr-viz
Intro music: "Books" by Minden: https://minden.bandcamp.com/track/books-2
- 1 participant
- 15 minutes
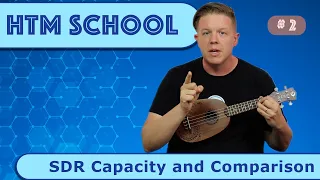
8 Apr 2016
Let's start at the very beginning! HTMs rely heavily on bit arrays, so here are the basics.
Intro music: "Books" by Minden: https://minden.bandcamp.com/track/books-2
Intro music: "Books" by Minden: https://minden.bandcamp.com/track/books-2
- 1 participant
- 12 minutes
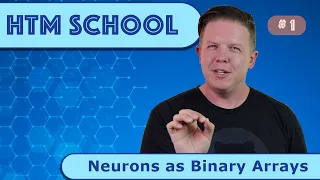
29 Oct 2014
"Sparse Distributed Representations: Our Brain's Data Structure"
Subutai Ahmad, VP Research, Numenta
Numenta Workshop Oct 2014 Redwood City CA
Subutai Ahmad, VP Research, Numenta
Numenta Workshop Oct 2014 Redwood City CA
- 7 participants
- 46 minutes
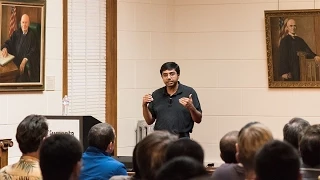
23 May 2012
In this screencast, Jeff Hawkins narrates the presentation he gave at a workshop called "From Data to Knowledge: Machine-Learning with Real-time and Streaming Applications." The workshop was held May 7-11, 2012 at the University of California, Berkeley.
Slides: http://www.numenta.com/htm-overview/05-08-2012-Berkeley.pdf
Abstract:
Sparse distributed representations appear to be the means by which brains encode information. They have several advantageous properties including the ability to encode semantic meaning. We have created a distributed memory system for learning sequences of sparse distribute representations. In addition we have created a means of encoding structured and unstructured data into sparse distributed representations. The resulting memory system learns in an on-line fashion making it suitable for high velocity data streams. We are currently applying it to commercially valuable data streams for prediction, classification, and anomaly detection In this talk I will describe this distributed memory system and illustrate how it can be used to build models and make predictions from data streams.
Live video recording of this presentation: http://www.youtube.com/watch?v=nfUT3UbYhjM
General information can be found at https://www.numenta.com, and technical details can be found in the CLA white paper at https://www.numenta.com/faq.html#cla_paper.
Slides: http://www.numenta.com/htm-overview/05-08-2012-Berkeley.pdf
Abstract:
Sparse distributed representations appear to be the means by which brains encode information. They have several advantageous properties including the ability to encode semantic meaning. We have created a distributed memory system for learning sequences of sparse distribute representations. In addition we have created a means of encoding structured and unstructured data into sparse distributed representations. The resulting memory system learns in an on-line fashion making it suitable for high velocity data streams. We are currently applying it to commercially valuable data streams for prediction, classification, and anomaly detection In this talk I will describe this distributed memory system and illustrate how it can be used to build models and make predictions from data streams.
Live video recording of this presentation: http://www.youtube.com/watch?v=nfUT3UbYhjM
General information can be found at https://www.numenta.com, and technical details can be found in the CLA white paper at https://www.numenta.com/faq.html#cla_paper.
- 1 participant
- 25 minutes
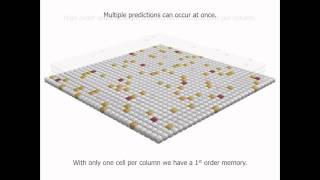