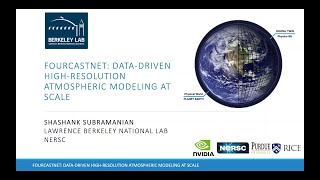
Description
NERSC Data Seminars Series: https://github.com/NERSC/data-seminars
Title:
FourCastNet: Data-driven, high-resolution atmosphere modeling at scale
Speaker:
Shashank Subramanian, Data & Analytics Services Group, National Energy Research Scientific Computing Center (NERSC), Lawrence Berkeley National Laboratory
Abstract:
We present FourCastNet, short for Fourier Forecasting Neural Network, a global data-driven weather forecasting model that provides accurate short to medium-range global predictions at 25km resolution. FourCastNet accurately forecasts high-resolution, fast-timescale variables such as the surface wind speed, total precipitation, and atmospheric water vapor with important implications for wind energy resource planning, predicting extreme weather events such as tropical cyclones and atmospheric rivers, as well as extreme precipitation. We compare the forecast skill of FourCastNet with archived operational IFS model forecasts and find that the forecast skill of our purely data-driven model is remarkably close to that of the IFS model for short to medium-range forecasts. FourCastNet generates a week-long forecast in less than 2 seconds, orders of magnitude faster than IFS, enabling the creation of inexpensive large-ensemble forecasts for improved probabilistic forecasting. Finally, our implementation is optimized and we present efficient scaling results on different supercomputing systems up to 3808 NVIDIA A100 GPUs, resulting in 80000 times faster time-to-solution relative to IFS, in inference.
Bio:
Shashank Subramanian is a NESAP for learning postdoctoral fellow with research interests in the intersection of high-performance scientific computing, deep learning, and physical sciences.
Host of Seminar:
Peter Harrington, Data & Analytics Services Group
National Energy Research Scientific Computing Center (NERSC)
Lawrence Berkeley National Laboratory